목적 : 새로운 샘플에서 가장 인접한 k개 샘플의 class에 따라 현재 class 분류
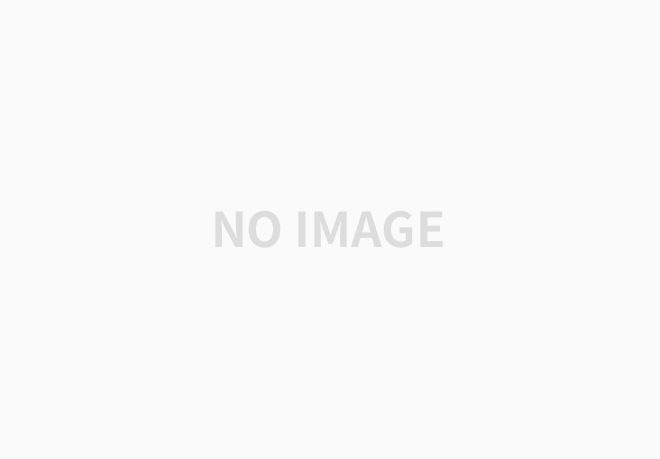
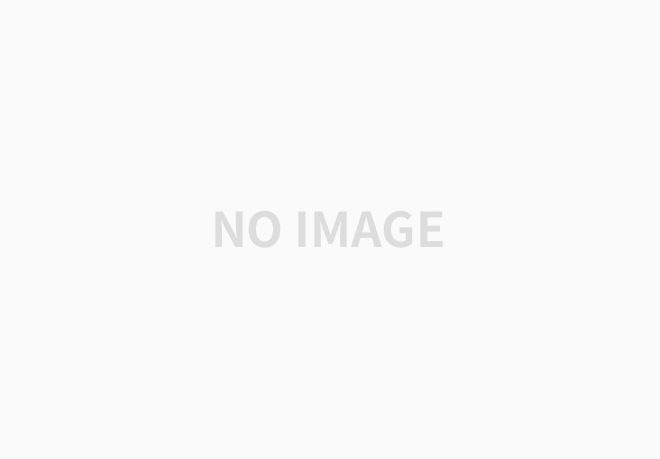
0. 3개의 class가 이미 training data로 들어와 있다.
1. distance 계산
2. 상위 4개를 뽑음
3. 어떤 class가 제일 많은지 확인 → 회식class도 끼워주세영~
▼ 패키지 선언
import pandas as pd
import numpy as np
import matplotlib.pyplot as plt
from sklearn.datasets import make_classification
from sklearn.model_selection import train_test_split
▼ Dataset 생성
X, y = make_classification(n_samples=500,
n_features=2,
n_classes=3,
n_clusters_per_class=1,
n_informative=2,
n_redundant=0,
random_state=40)
plt.scatter(X[:, 0], X[:, 1], marker='o', c=y, s=100, edgecolor="k", linewidth=1)
plt.xlabel("x_1")
plt.ylabel("x_2")
plt.show()
# Training/Testing Dataset 분리 (80:20)
X_train, X_test, y_train, y_test = train_test_split(X, y, test_size=0.2, random_state=1234)
print("Training samples: ", len(X_train))
print("Testing samples: ", len(X_test))
더보기
Training samples: 400
Testing samples: 100
▼ Euclidian Distance 함수, KNN 모델 작성
def L2_distance(x1, x2):
return np.sqrt(np.sum((x1 - x2) ** 2))
class KNN:
def __init__(self, k=3):
# initialization
self.k = k
def fit(self, X, y):
# Storage training datas
self.X_train = X
self.y_train = y
def predict(self, X):
# Prediction
y_pred = []
for X_i in X:
distance = [L2_distance(X_i, X_train_i) for X_train_i in self.X_train]
k_idx = np.argsort(distance) [:self.k]
k_labels = [self.y_train[i] for i in k_idx]
most_class = max(k_labels, key = k_labels.count)
y_pred.append(most_class)
return y_pred
▼ 예측 및 성능 평가
model = KNN()
model.fit(X_train, y_train)
y_pred = model.predict(X_test)
accuracy = np.sum(y_pred == y_test) / len(y_test)
print(accuracy)
더보기
0.81
▼ 예측 결과 시각화
plt.figure(figsize=(12,6))
plt.subplot(1,2,1)
plt.title("label") # label : 정답 데이터
plt.scatter(X_test[:, 0], X_test[:, 1], marker='o', c=y_test, s=100, edgecolor="k", linewidth=1)
plt.subplot(1,2,2)
plt.title("prediction") # prediction : 예측 값
plt.scatter(X_test[:, 0], X_test[:, 1], marker='o', c=y_test, s=100, edgecolor="k", linewidth=1)
plt.show
▼ 데이터 특성에 따른 고려사항
X, y = make_classification(n_samples=500,
n_features=2,
n_classes=4,
n_clusters_per_class=1,
n_informative=2,
n_redundant=0,
random_state=40)
X[:, 0] = X[:, 0] * 0.1 - 100
X[:, 1] = X[:, 1] * 100 + 120
plt.scatter(X[:, 0], X[:, 1], marker='o', c=y, s=100, edgecolor="k", linewidth=1)
plt.xlabel("x_1")
plt.ylabel("x_2")
plt.show()
'머신러닝' 카테고리의 다른 글
K-Nearest Neighbor (KNN) (0) | 2023.12.18 |
---|---|
Entropy (0) | 2023.12.18 |
Support Vector Machine : Gradient Decent Method (GD) (1) | 2023.12.18 |
Support Vector Machine : Quadratic Programming(2차 계획법) (0) | 2023.12.18 |
MNIST Classification using SLP, MLP (1) | 2023.12.17 |